- General Intelligence
- Posts
- Issue #1: Improving Medical Image Understanding
Issue #1: Improving Medical Image Understanding
Plus advancements in rain nowcasting, speech recognition without web-scale data, generating synthetic data at scale
1
In this round of research papers, we highlight some cool advancements in the application of AI models. We can predict short-term rain forecasts, improve the quality of medical images, create massive amounts of synthetic data, and get more accurate speech recognition with less data.
🌧️ GPTCast uses LLM techniques to predict short-term rain forecasts.
The paper presents GPTCast, a new AI method for predicting rain. Inspired by how language models like GPT work, it uses radar images to forecast precipitation. This model is trained on six years of weather data from Italy and shows better performance in predicting rain compared to existing methods, providing more accurate and realistic weather forecasts. Link to arxiv.
💬 Accurate Speech Recognition & Translation without Web-Scale Data
Canary, a model that excels in speech recognition and translation for multiple languages using significantly less training data than usual. It achieves state-of-the-art performance through a new model architecture, synthetic data generation, and advanced training techniques. This efficient approach suggests that high-quality AI language models can be developed without relying on massive amounts of internet data. Link to arxiv.
🩻 Improving Medical Image Understanding with PubMed
This paper discusses the development of an advanced AI model that combines visual and textual medical knowledge to improve medical image understanding. The authors created a new dataset called PubMedVision with 1.3 million medical image-question-answer pairs by refining existing medical data. This dataset allows their model, HuatuoGPT-Vision, to outperform other open-source models in interpreting and generating accurate medical information from images. Link to arxiv
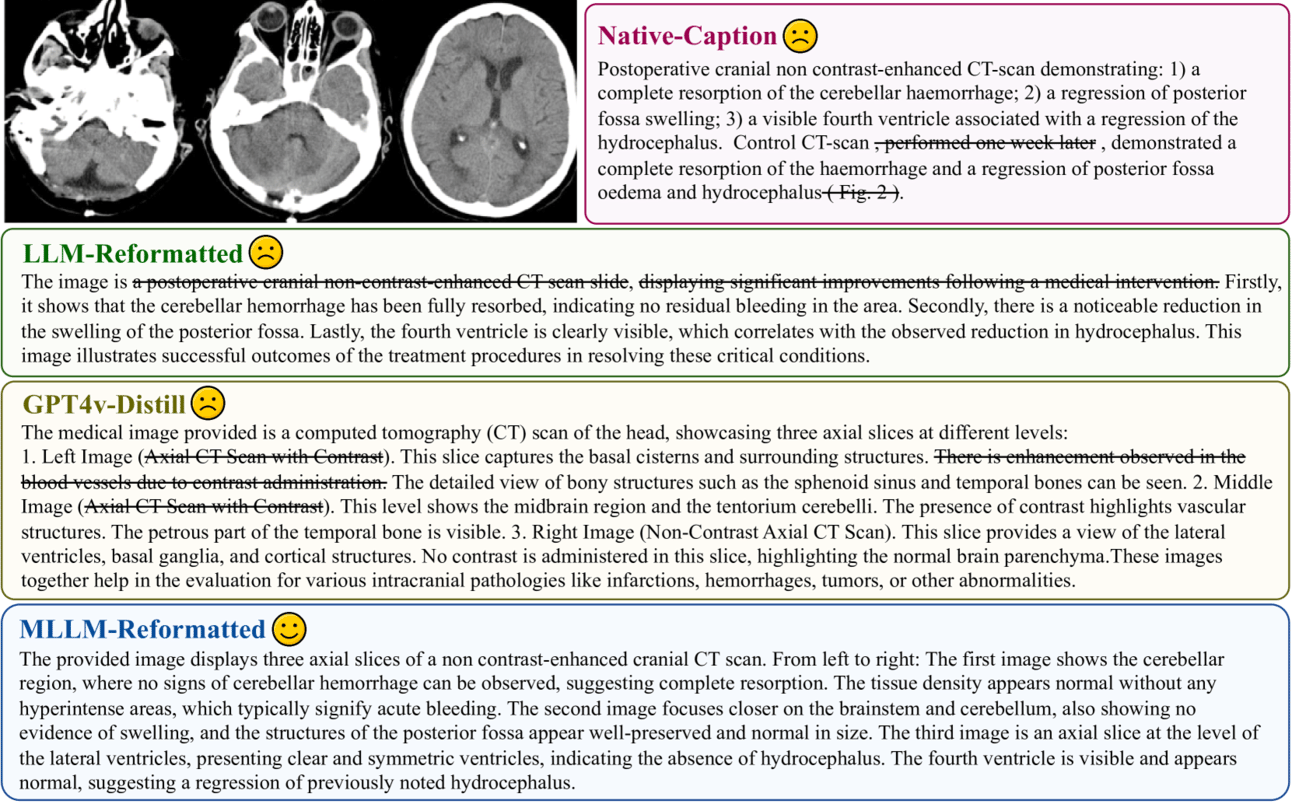
👥 Using 1 Billion Personas to Create Synthetic Data at Scale
Tencent Researcher presents a method for generating large-scale synthetic data using AI models that mimic the perspectives of one billion diverse personas. This approach, called Persona Hub, creates a vast and varied dataset to train AI for tasks like solving math problems, generating user prompts, and creating game characters. The authors highlight how this scalable and versatile method could significantly impact AI development and applications by providing more comprehensive and diverse training data. Link to arxiv
